
Structured data acquisition is a common task that is widely performed in biomedicine. The current versions of DMO, ADMO and ADMO-plus are freely available at. Finally, we expand the model using elements from other resources, such as clinical features contained in the AD Ontology, resulting in an enriched model called ADMO-plus. Furthermore, we show that it can store and manage multi-omics data. We demonstrate that it allows one to deal with issues related to redundancy, naming, consistency, process classification and pathway relationships. Specifically, we use it to drive the conversion of AlzPathway, a disease map devoted to AD, into a formal ontology: Alzheimer DMO. We then propose to apply DMO to Alzheimer's disease (AD). We introduce the Disease Map Ontology (DMO), an ontological upper model based on systems biology terms. Ontology is an adequate framework to overcome this limitation, through their axiomatic definitions and logical reasoning properties. However, the expressiveness of disease maps could be increased to help in avoiding ambiguities and misinterpretations and to reinforce their interoperability with other knowledge resources. Disease maps provide curated knowledge about disorders' pathophysiology at the molecular level adapted to omics measurements. The integration and interpretation of such data pose major challenges, calling for adequate knowledge models. Omics technologies offer great promises for improving our understanding of diseases. PhenGenVar is freely accessible and is available at. Therefore, PhenGenVar can be used to diagnose a patient’s disease. As a result, we were able to identify several genomic variations, including single-nucleotide polymorphism, insertions, and deletions in specific gene regions. To test this program, we analyzed the whole-genome sequencing data of an anonymous person from the 1000 human genome project data.
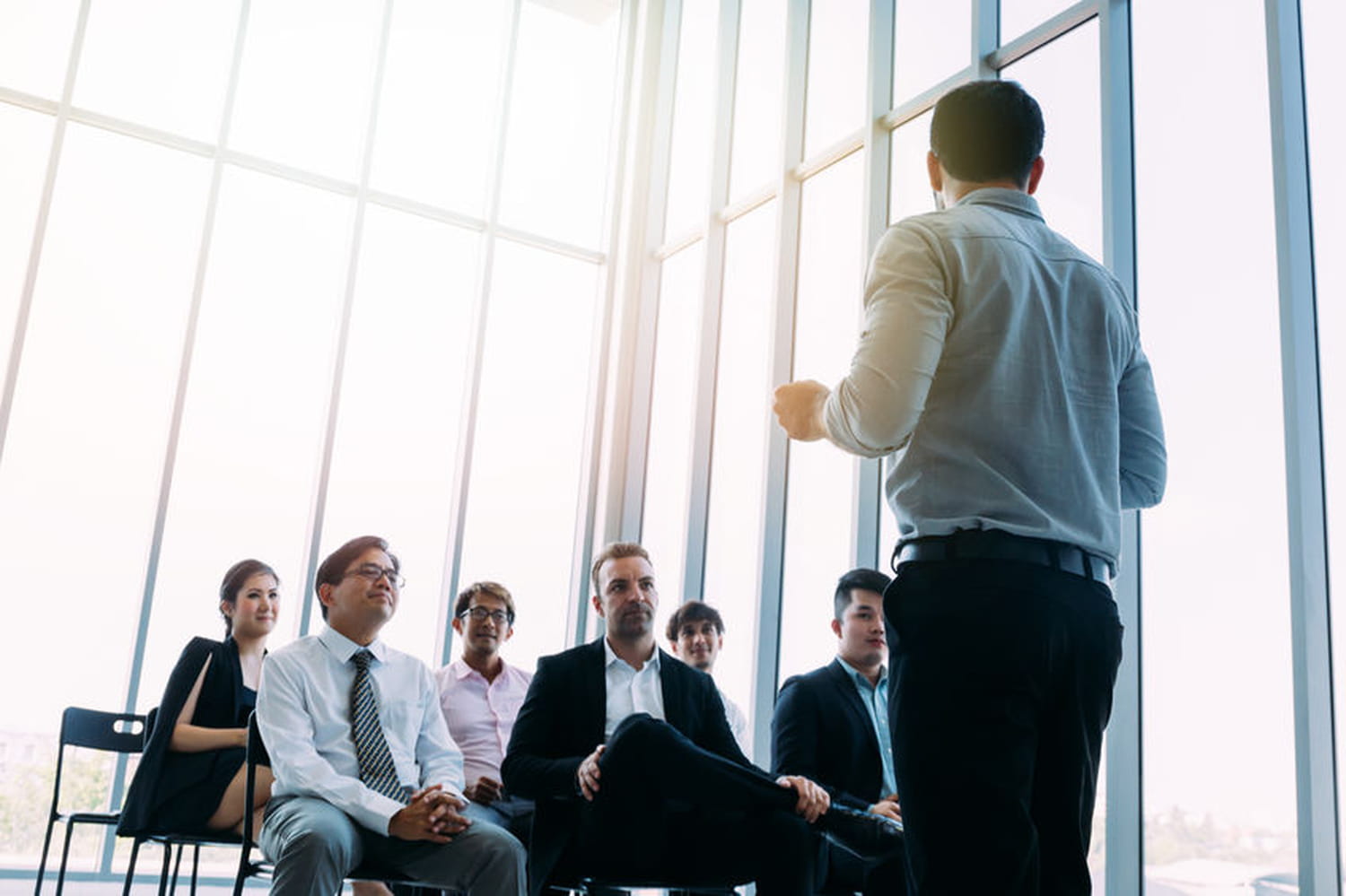
This allows for flexible filtering and browsing of variants of the disease and phenotype-associated genes. In response to this demand, we developed a Phenotype to Genotype Variation program (PhenGenVar), which is a user-friendly interface for monitoring the variations in a gene of interest for molecular diagnosis. Although many bioinformatics tools have been developed to analyze the genetic variations from the individual patient’s NGS data, it is still challenging to develop user-friendly programs for clinical physicians who do not have bioinformatics programing skills to diagnose a patient’s disease using the genomic data. These breakthroughs paved the way for the clinical diagnosis and precise treatment of patients’ diseases. Furthermore, patients’ genetic variations related to a specific phenotype can also be identified by analyzing their genomic information. At the population level, biomedical studies have identified human diseases and phenotype-associated genetic variations using NGS technology, such as whole-genome sequencing, exome sequencing, and gene panel sequencing. Precision medicine has been revolutionized by the advent of high-throughput next-generation sequencing (NGS) technology and development of various bioinformatic analysis tools for large-scale NGS big data.
